How to Build Trust in AI to Scale Adoption
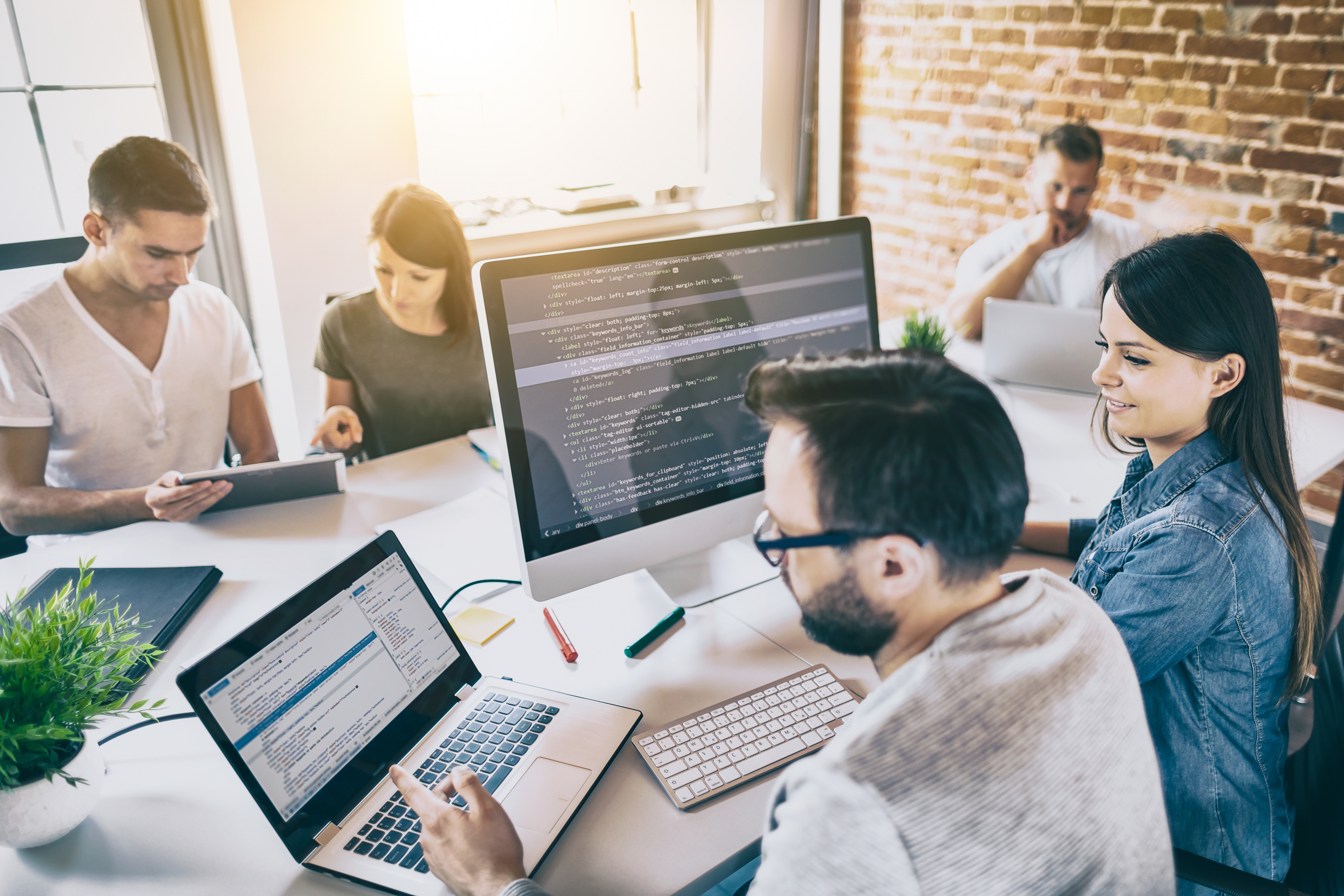
Financial services firms are utilizing AI and machine learning to improve their operations and offer more relevant and personalized solutions to consumers, but studies consistently show the need to build trust in AI to scale adoption. The pandemic has exacerbated the need for AI, with 52 percent of companies stating that they accelerated their AI adoption plans and many more planning to follow suit in the coming years. Though the public's AI trust has been on the rise, there are still some lingering concerns about the ability of AI-based solutions to rank over human-facing offerings.
As competition in the financial services field grows and consumers become more selective, firms must offer more personalized solutions faster to remain competitive. 86 percent of businesses who have implemented AI believe that AI became a mainstream technology for their company in 2021, and adopting AI into a company's operations improves customer experience and efficiency. However, a study by IBM reveals that 63% of respondents cited a lack of technical skills as a leading barrier to AI implementation.
Most organizations do not have a dedicated data science team or the necessary AI experts, leading business owners to take responsibility for AI projects. Without the knowledge or tools to help them understand how AI and machine learning systems actually work, business owners struggle to take experiments out of the lab and into production. According to MIT, 82 percent of organizations surveyed stated that they had failed to adopt AI beyond pilot or proof-of-concept, suggesting that there is little confidence in AI's ability.
Trust in AI
Given the rapid pace of innovation throughout this century, it is no surprise that consumers are not entirely familiar with the latest technological advances. AI trust is not a guarantee as the public struggles to fully understand the technology and be confident in the quality of the solution they're receiving. Consumers want to ensure they're getting a comparable service when provided by AI to when humans offer it.
AI trust will be instrumental to a company's ability to integrate it into its operations. While AI and machine learning can make a company more efficient, see cost savings, and improve the customer experience, there is still a bridge to gap regarding people's trust in these systems and the willingness to accept solutions and recommendations from AI systems over humans.
Without a good understanding of how companies utilize AI, consumers may be wary of accepting an AI or machine learning solution. Not to mention that 81 percent of business leaders report that they do not fully understand the data and infrastructure needed for AI. So, even though AI solutions can benefit both firms and consumers, the lack of transparency and mistrust in AI can account for why financial services firms have been slower to adopt AI.
As business leaders and consumers become more familiar with AI solutions, it will be easier for companies to accelerate AI adoption. But how can we get there? Here are four ways to build trust in AI to accelerate adoption.
4 Ways to Build Trust in AI to Scale Adoption
Although there is still some hesitancy regarding AI-based solutions, there are various ways that financial services firms can help improve this sentiment. The more familiar business leaders and consumers are with AI and machine learning, the more they will feel comfortable and have increased trust with AI-based recommendations and solutions, allowing businesses to adopt AI more rapidly into their operations.
Explaining AI
The lack of understanding of AI partly explains why there is distrust in AI and hesitancy for adoption. According to Venture Beat, 64 percent of executives can't explain how their AI models make decisions. Given that it's a complex technology, many non-experts are uncertain of how AI works, thus are unable to have confidence in the system and how it generates responses.
The more people can understand the inner workings of AI and machine learning, the more they will have trust in AI and be more accessible for firms to implement. Both business leaders and consumers could benefit from better knowledge about this technology and how companies plan to use it in their operations. Technology teams and decision-makers can help their teams understand AI better by providing AI education sessions and content, creating a plan around AI implementation, and showing the potential ROI benefits of adopting AI.
By helping a company's decision-makers, like CEOs, the compliance team, and the board of directors, better understand how AI can help their business and improve efficiency and profitability, the quicker they will want to adopt AI and machine learning solutions. With increased transparency and confidence in how it works, businesses' trust in AI grows and is more quickly adopted.
Preventing bias from data
Though a cutting-edge technology, AI isn't always perfect and still has improvements regarding its level of bias in financial decision-making. In the financial industry, AI is now used to determine who qualifies for a bank loan, recommend insurance quotes, detect fraud, and perform risk management, among other functions. Given the importance of these decisions on both consumers and firms, AI algorithms must produce accurate and fair recommendations without bias.
People want to know they are receiving unbiased recommendations from AI systems, so removing the bias in these programs is vital for building AI trust. Not only is an inaccurate and biased recommendation unfair to consumers, but it can also be costly for firms who may face legal disputes if an AI model is not correct and leads to erroneous decisions or recommendations.
A transparent AI system that can show how it made a decision would help business leaders and consumers see justified reasoning behind its recommendations. Building trust in AI is an issue companies will need to address, so better transparency into the possible biases that AI systems can have and how that's being mitigated is one way of helping consumers and teams feel more confident in its use. With quality data, well-trained models, and frequently-tested systems, businesses and consumers can ensure that the AI is working correctly and without bias and feel more comfortable adopting it into their operations.
Preventing Drifts in AI Models
Built on an algorithm to solve a specific problem, AI models continually adapt to the data sets input into it. This is very effective for AI to learn the similarities and possible outliers of a data set, but it is not entirely foolproof. AI models are only as good as the data it analyzes, and data quality is essential to keeping AI systems accurate and unbiased. A potential threat to using AI in a business is avoiding drift, which is when AI model analyzes data that differs from what it was originally trained to evaluate.
Over time, AI and machine learning that are trained for a specific purpose may have accidental biases against data sets that differ from the primary goal. For example, an AI system built to evaluate male college applicants only may have an inherent bias against female applicants.
Adequate testing is necessary for utilizing an AI system in your business. Without frequent checks into how accurate the AI or machine learning is, a firm risks using a less reliable and inaccurate model. Firms can establish trust with their clients by ensuring that frequent testing is a standard for operations and that possible biases and drift are being monitored, making it easier for companies to ramp up adoption.
AI Infrastructure
Aside from having a transparent model and frequent testing that helps prevent drift and bias, financial services firms must have sufficient infrastructure and architecture to ensure that their AI models are successful. In order to scale AI adoption within the business, the infrastructure must support AI use on a high level and promote AI trust.
As previously mentioned, data quality is a primary key to running an accurate and reliable AI system. Having a place to securely store large amounts of data should be a priority for financial services firms to build AI trust among consumers and business leaders. Different stages of an AI system include ingesting, preparing, training, and inference, and each step requires its own standard for data storage.
With the proper infrastructure in place, financial services firms can feel confident that they provide quality data for the AI systems to work effectively, and consumers can trust that the output will be fair and relevant. So, to successfully ramp up AI adoption into its operations, a company must have the proper environment and architecture in place.
Using No-Code AI to Accelerate AI Adoption
Financial services firms must utilize AI to make their systems more efficient, accurate, and secure to stay competitive in today's landscape. As business leaders and consumers grow more comfortable and familiar with AI and machine learning, AI and trust will become less of a concern for firms and more accessible for them to accelerate AI adoption.
Financial services firms don't need employees to code or hire an expert to help adopt AI into their operations. Using a no-code AI solution, like Accern, is a simple way for firms to develop their offerings, stay up-to-date with technology, and implement AI into their operations. A no-code AI platform makes it easy for executives and employees alike to understand how AI works and build AI models. Furthermore, a no-code AI platform, like Accern, offers a SaaS and secure deployment options to ensure data security and help companies manage AI infrastructure. See how easy it is to accelerate AI adoption by requesting a free demo with Accern today.