How ESG Impacts Pricing and Lending Decisions
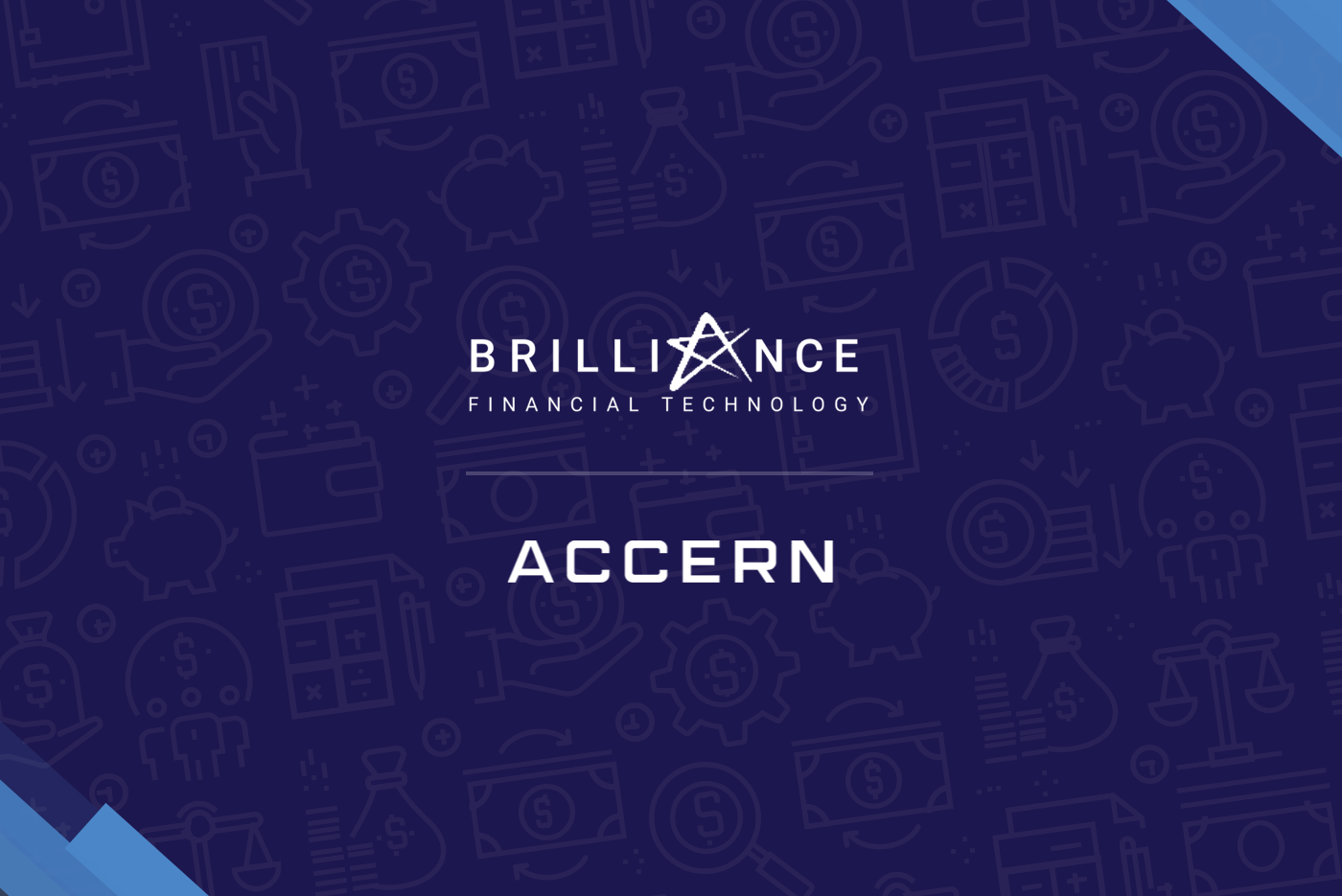
By: Riyaz Nakhooda, VP of Strategic Partnerships at Accern in collaboration with Mark Gu Chen, Head of Product Management at Brilliance Financial Technology
It’s difficult to open a paper or watch the news without seeing how events like climate change, deforestation and the actions of businesses are impacting society. Due to this ubiquity, society now demands companies to conduct business with strong Environmental, Social and Governance (ESG) practices. Companies that can prove their ESG practices will have a greater positive impact on society and remain competitive over those that fail to be ESG compliant. This blog post will cover how ESG impacts pricing and lending decisions.
According to a study by S&P Global, how a company performs on sustainability factors relevant to their industry can be an important determinant of financial performance. According to the above chart, a majority of studies have found that firms that pay attention to ESG concerns have a positive return on equity (McKinsey). It’s no wonder that financial services firms, and, as the main focus of this piece, banks, are increasingly taking into account and weighing a company’s ESG practices in their investment, risk management, and lending activities.
When it comes to lending, a firm’s ESG impact not only manifests in its financial performance - it’s also a key risk factor. Companies operating in non-sustainable industries or with poor ESG practices are a riskier proposition and this should be taken into consideration when banks enter into long term relationships with their customers.
However, many banks only view ESG factors as part of credit approval or origination process, after an indicative offer has already been made with pricing tentatively agreed upon. In this article we’ll explore why this is problematic and how and where ESG should be incorporated in the lending process.
Bringing Real-Time Pricing Decisions to the Forefront
Until recently, pricing decisions for commercial lending were almost exclusively made by specialists in the middle and back office. The complex nature of commercial deals, the varying profitability model, their associated credit risk data, as well as the non-linear business processes were often cited as reasons why real-time pricing decisioning was not possible for the front line.
Today, however, almost every bank’s digital transformation program includes pricing and profitability analysis for bankers as a key part of a banker's journey, often at great effort and cost.
So what happened? Why are banks willing to invest in this area now? Having worked closely with many Tier 1 and 2 global banks, we know that bringing pricing decisions into the hands of relationship managers on the frontlines not only improves the bank’s operational efficiency, it also helps the bank in many other strategic areas. For example, banks can apply consistent pricing across the organization, consider holistic relationship profitability with future opportunities, and most relevant for this article, allow the frontline to structure competitive and profitable deals with the latest credit risk data in real-time.
Applying This Lesson to ESG
It is absolutely crucial for banks to stay on top of the latest data available, be it Credit, ESG, etc., allowing a bank to offer the best price against the risks they are taking. Since ESG is dynamic and reflects the most current state of a customer, it makes sense that ESG data be made available in real-time to the frontline. Relationship managers then would be able to use this information during initial negotiations with customers, using pricing as a carrot or stick, all before credit review or the origination process. With AI/ML and cloud computing, it’s now possible to present structured ESG data from a wide array of sources to the front-line in real-time.
For the frontline, rather than treating ESG as yet another process to complete, key ESG data points could be presented intuitively to the bankers to help them secure deals. For example, timely ESG alerts relevant to a particular industry can trigger conversations. Additionally, ESG sentiment with a relationship can potentially affect the outcome of an opportunity. This can be both positive or negative, resulting in rate reductions or premiums for certain industries.
Why ESG Doesn’t Belong Elsewhere in the Lending Process
Currently, banks store ESG data in various systems, applying them at different stages of the lending process. Here are two common systems:
1. CRM: Because CRMs provide overviews of relationships for bankers, some might feel this is also the natural place to maintain ESG information.
Why it’s not ideal: Whilst it’s true that a great banker pays attention to all of his/her relationships all the time, in reality most bankers will only pay close attention to a relationship when there is an opportunity. There just isn’t enough time and sales incentives are not designed to allow them to look at ESG activities for all of their relationships. However, when there is a new opportunity - pricing is always the first point of discussion. If pricing is not competitive, the banker will be unlikely to pursue the opportunity. So this is precisely the moment that ESG data should be provided to the frontline- because they will pay close attention to important factors that can impact the success of a deal.
2. Origination Platform (Credit Approval): Banks typically consider the credit approval process as the control gate for deal approval, so it might make sense to group ESG information together with credit decisions.
Why it’s not ideal: ESG information needs to be presented during the initial conversation and deal structuring / pricing process. Banks should be able to develop sophisticated rules based on various ESG factors of the relationships, shaping both the type of product offered, the terms of the products, and pricing outcomes. So while having ESG reviewed during the credit approval process can help banks avoid bad customers, the fact that it takes place after commercial decisioning or initial offers means the bank might’ve already lost opportunities with good customers by offering uncompetitive pricing.
What to consider when building an ESG Assessment Framework
While the reasons to incorporate ESG into lending decisions may be clear, banks still must grapple with selecting reliable tools, frameworks and data sources to identify and quantify the materiality impacts.
They must first consider the appropriate ESG lens they want to apply and observe a company's ESG practices and its impact on society and the environment. This may include using industry frameworks such as MSCI, SASB, SDG or internal ratings frameworks they’ve developed. Furthermore, it is equally important to assess a company’s stance on ESG controversies such as racial injustice, mining incidents and other ongoing controversies.
Once an appropriate lens is determined, organizations must gather the correct data to support an accurate ESG analysis. It is often difficult to get granular, underlying data for individual companies to support an ESG assessment. This is due to an inconsistency in the requirements of how organizations are to publish their ESG information as well as the way the information is typically spread across various reports. The data issue is further compounded due to the fact that many companies do not have the resources to provide adequate ESG information since they don’t have dedicated sustainability teams in the first place.
Additionally, many organizations prefer to not disclose issues that may impact their reputation. This is often seen with social issues like diversity where companies are reluctant to share demographics in their data, and for some organizations sharing that information can even be illegal. In fact, S&P states that only about a third of the companies assessed globally provide a breakdown of their workforce by race and ethnicity on their own or to the public.
In addition to the challenges listed above, there is also a timing and frequency issue at which companies update their ESG information. On average, companies report updated metrics on a quarterly or yearly basis, which is too infrequent when the applications require a real-time analysis. In fact, according to a 2020 report released by the US Government Accountability Office (GAO), investors find that ESG disclosures “lack detail, consistency, and comparability.”
Overcoming Inconsistent or Non-Existent ESG Reports
Although there are several challenges and complexities in setting up a robust ESG assessment framework, there are solutions to help banks and financial services organizations.
A foundational step is to address the data issue by assessing content beyond CSR, annual reports and sustainability reports, in order to include non-traditional sources such as global and local news, trade journals, social media, investor presentations, earning transcripts, surveys, reviews and other corporate filings.
By expanding the sources analyzed, it helps to address a number of other stated challenges. For example, we will often find mentions of social issues around a company mentioned in the news and various media outlets. Additionally, we can now assess the company’s ESG practices with more frequently reported data. Finally, by expanding our data sources we can potentially discover ESG information on both public and private companies that do not have the teams in place to publish their disclosure.
While expanding the set of content sources is a great way to better understand an organization’s ESG practices, how is one able to actually do this without an army of analysts that can comb through all the data? Thanks to recent advancements in AI and machine learning, we are now able to construct models that can analyze large volumes of documents and automatically identify, extract and quantify a company’s ESG practices - no army of analysts required.
Additionally, a good AI model should enable organizations to adapt its taxonomy to identify practices and controversies based on the specific lens that the bank or financial service organization would like to employ for its ESG Assessment.
Finally, the output of the models can easily be visualized to help in the quick identification of areas of risk for an individual company, trends over time or a comparison to a peer group (see an example below, using the Accern platform).
In Conclusion
Using the latest AI/ML techniques, complex ESG data can now be utilized and intuitively incorporated into the deal structuring and pricing process. This will allow Relationship Managers and Bankers to price deals using the latest ESG data during the client negotiation process. This shift will allow banks to originate deals with both credit risk and ESG risk in mind. As this step is a key part of every banker’s focus, it is also the most effective way to create ESG cultural awareness within banks.
Finally, considering recent events occurring globally, such as burning forests, rising shorelines, and even the Gamestop controversy, it is clear that timely ESG data should be presented to the frontlines, and what more effective way than to do this than making it available as part of the pricing & commercial engagement process?
Want to learn more about how effectively to collect & incorporate ESG factors into your end to end process? You can learn more about Accern and Brilliance below.
About Brilliance
Brilliance Financial Technology has delivered digital pricing and profitability solutions to banks in 50 countries, servicing over 25,000 relationship managers. DPX, our 4th generation cloud-native platform, uniquely combines pricing, rates, and profitability management to help banks thrive in today’s dynamic market conditions. DPX improves your profits, ensures your bank’s regulatory compliance, and delivers pricing transparency across your organization. www.bxfin.com
About Accern
Accern enhances artificial intelligence (AI) workflows for financial services enterprises with a no-code AI platform. Researchers, business analysts, data science teams, and developers use Accern to build and deploy AI use cases powered by adaptive natural language processing (NLP) and forecasting features. The results are that companies cut costs, generate better risk and investment insights, and experience a 24x productivity gain with our smart insights. Allianz, IBM, and Jefferies are utilizing Accern to accelerate innovation. For more information on how we can accelerate artificial intelligence adoption for your organization, visit accern.com