How Artificial Intelligence Is Used In Asset Management
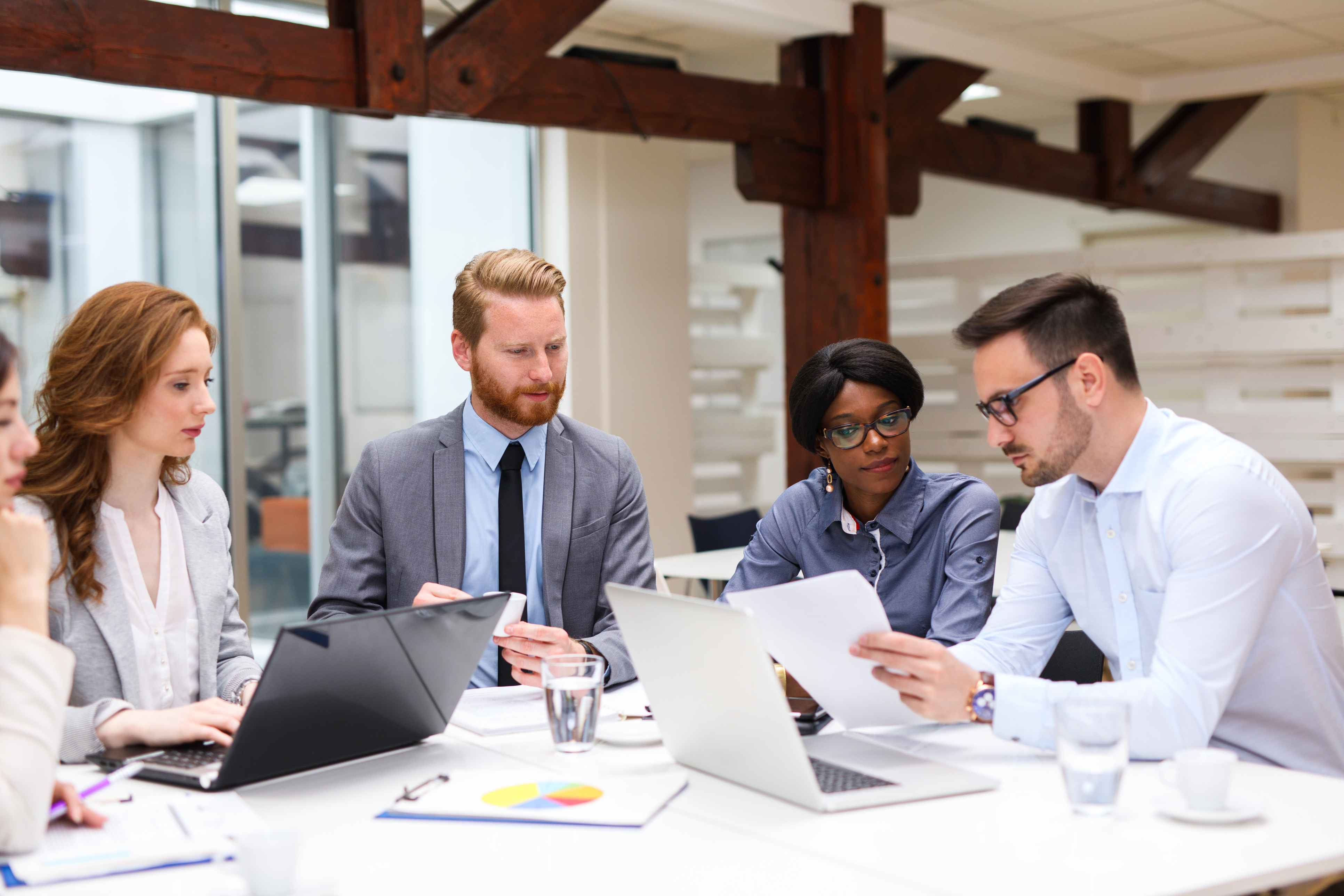
The volume and velocity of new data generated is an obstacle that many analysts face. Fundamental analysts spend hours manually attempting to identify specific points within hundreds of data sources. The asset management industry is realizing the transformative impact that artificial intelligence has within the front, middle, and back operations. Read on to find out how artificial intelligence is used in the asset management industry today.
Artificial intelligence enables fundamental analysts to research and extract large quantities of information quickly for asset managers to analyze and turn into actionable insights. In order to capture all of the data around specific companies, services, products, etc, analysts search through hundreds of sources and consistently monitor the news. However, new data is constantly generated, making human error within research inevitable. The results of inaccurate or out-dated data can lead asset managers in making the wrong decisions and cost an asset management firm thousands to hundreds of thousands in revenue depending on the investment.
Artificial intelligence's benefits to the asset management industry include its ability to minimize human error. This is increasingly recognized within the financial services industry. Within asset management, AI is transforming the workflows of analysts, data scientists and engineers, and asset managers through natural language processing (NLP) techniques.
NLP is a subset of artificial intelligence that enables computers to understand text and audio data in the same way that humans can- with the human language. It has gained even more recognition in recent years and is transforming the way that financial services work. When NLP is applied to unstructured text, specific words from data can be detected and the most relevant facts can be extracted. The AI can be paired with AutoML (automated machine learning) to automate the process of searching for specific key words and extracting relevant information. Analysts can then obtain specific data points in real-time without having to be stuck to a monitor scrolling through endless sources. Read more about NLP and what the use cases of NLP in finance are.
Source: Medium
Data Mining for Business Analytics
Asset management firms that know how to utilize AI to clean data will remain competitive and experience the results of drawing quicker and more accurate insights. NLP enables analysts within asset management firms to collect and summarize all of the necessary information from large amounts of data and reveal potential next steps for asset managers to implement in their investment decisions. With NLP, the AI tool can quickly extract the most relevant insights from various sources to aid asset managers in their investment decisions.
Big Data, which is the collection of clean data from structured and unstructured data sources, comes from analyzing massive amounts of unstructured documents. Unstructured data comes in different formats and with a lot of noise. Spreadsheets, audio transcripts, videos, and images are all forms of unstructured data. With the large variety of data, it is nearly impossible for analysts to manually identify and extract the information needed to aid in investment decisions at the right time. NLP can help clean through the data by searching sources, detecting relevant information, and converting it into an understandable format.
The velocity at which data is generated is increasing by the second as digital tools are evolving and new tools are being introduced. AI can enable financial analysts to gather the main important facts from data, and AutoML can increase productivity by automating the process. In turn these data mining insights can be used for business analytics to help asset managers create actionable next steps in real time.
Ways Artificial Intelligence is Used in Asset Management
Investment Research
Researching a company for investments is often difficult and time consuming. If corporations were transparent about all of their policies, actions, employee engagement and turnover, sustainable impact initiatives, and more, it would be much easier. However, the fact that corporations are not forced to disclose certain information to the public makes it difficult for investment, portfolio, and asset managers to understand and predict the long-term financial impact of a company's actions.
Financial analysts can spend hours and even days researching through various data sources and extracting the right information to determine future financial performance. However, by the time analysts find information, new information is generated. The manual research processes often causes long hours of digging through noise in data, and can make it prone to human error.
In order for asset managers to identify clear next steps to take with their portfolios, they need to have a well-rounded view on the company, its products and/or services, the sentiment around the company, its interactions with competitors and consumers, and more. Any negative or positive news, review, social post, etc can impact a company's financial direction, especially when dealing with large corporations. For example, most corporations aren't forced to disclose employee diversity. However, if a corporation hasn't disclosed that information but the truth about a lack of diversity within a company's hiring practices is revealed, that can affect the company's reputation and cause a negative stock price movement.
With artificial intelligence and machine learning, analysts and data scientists can take the noise out of data mining, automate the research and extraction process, and perform sentiment and relevance analysis on social media, news, and more. Sentiment analysis is used to determine the overall sentiment from the data text and source about the company and its service or product while relevance analysis is used to determine the relevance of the news in relation to the financial team's specific goals.
Source: Deloitte
Portfolio Construction
With data mining and business analytics, financial teams can identify patterns and build AI models around them. Data scientists and engineers can use AI to create automated screening factors on specific events like mergers and acquisitions, interest rates, trade wars, and natural disasters. By seeing how past events have impacted a company's portfolio, the information can be used to predict future events on a company's stock price.
For example, if we analyze the impact of the historic pandemic, SARS, on the retail industry, we can predict the likelihood of how COVID-19 will impact the retail industry again given similar circumstances. Additionally, by analyzing previous natural disasters' impacts on specific industries, we can predict how future disasters will impact those same industries, and so on.
Another way that NLP can help with portfolio construction is to identify new investment opportunities, boost returns, and manage risk. Since corporations aren't so transparent about many of their actual practices, NLP can find information by digging deeper than surface news. For example, it's nearly impossible to tell if a company is really following its ESG mandate. NLP can be used to monitor a company’s ESG compliance by searching through thousands of documents, tagging unstructured data, and revealing hidden information for analysts to review.
Accern's No-Code AI Solution for Asset Management Firms
With the advancement of AI and ML tools, we were able to develop a no-code AI platform at Accern, enabling financial analysts and data scientists and engineers to use artificial intelligence without having to code. The goal is for financial teams to easily implement AI and ML within their data sourcing processes so that efficiency and productivity is enhanced across the value chain.
With the growth in unstructured data, asset management firms that can quickly extract information to execute well-informed investment decisions at scale will have the most leverage in a competitive landscape. Researchers and data scientists and engineers within asset management firms can deploy ready-made NLP use cases as well as build custom NLP models. With its focus on empowering and easing workflows for data scientists and engineers and financial analysts, Accern's platform can be used for sentiment analysis, relevance analysis, NER (named entity recognition), foreign language translations, and text summarization.
With billions of data sources on the web, it can be hard for researchers to monitor multiple web pages in real-time. Accern solved this by partnering with major financial news providers like Dow Jones, FactSet, Thomas Reuters, Bloomberg and others to bring large amounts of clean data into our platform for researchers and data scientists and engineers to access in one place. The specific datasets users can access from our platform include Dow Jones Institutional Newswire, Morningstar Equity Research, and various Naviga datasets. After data is analyzed, you can view detailed and visual reports through a business intelligence (BI) tool like Kibana. The Kibana dashboard enables you to build your own visualization through charts, plots, and graphs on top of large amounts of data.
One of the ways in which AI adoption has taken place within asset management firms is by integrating artificial intelligence in firms' CRM systems. This enables firms to keep track of customer notes, emails, meetings, and more. Accern enables financial teams to upload data from their own CRM system, excel spreadsheet, Word doc, PowerPoint, and PDF to build NLP use cases. Visit The Benefit of Integrating Artificial Intelligence in CRM Systems to learn more about why financial firms are integrating AI with their CRM systems.
The use of AI within the asset management industry is growing but there is still much work to be done before we can say most asset management firms have implemented artificial intelligence. The time consumption, cost factors, and expertise needed in creating and training AI models are obstacles to AI adoption. However, with no-code AI, we are one step closer to enabling financial services enterprises to adopt artificial intelligence quicker.
For more information on how artificial intelligence can be used in asset management, download our free e-book today.
About Accern
Accern is a no-code AI platform that provides an end-to-end data science process that enables data scientists at financial organizations to easily build models that uncover actionable findings from structured and unstructured data. With Accern, you can automate processes, find additional value in your data, and inform better business decisions- faster and more accurately than before. For more information on how we can accelerate artificial intelligence adoption for your organization, visit accern.com