How AI is Shaping the Microfinance Sector
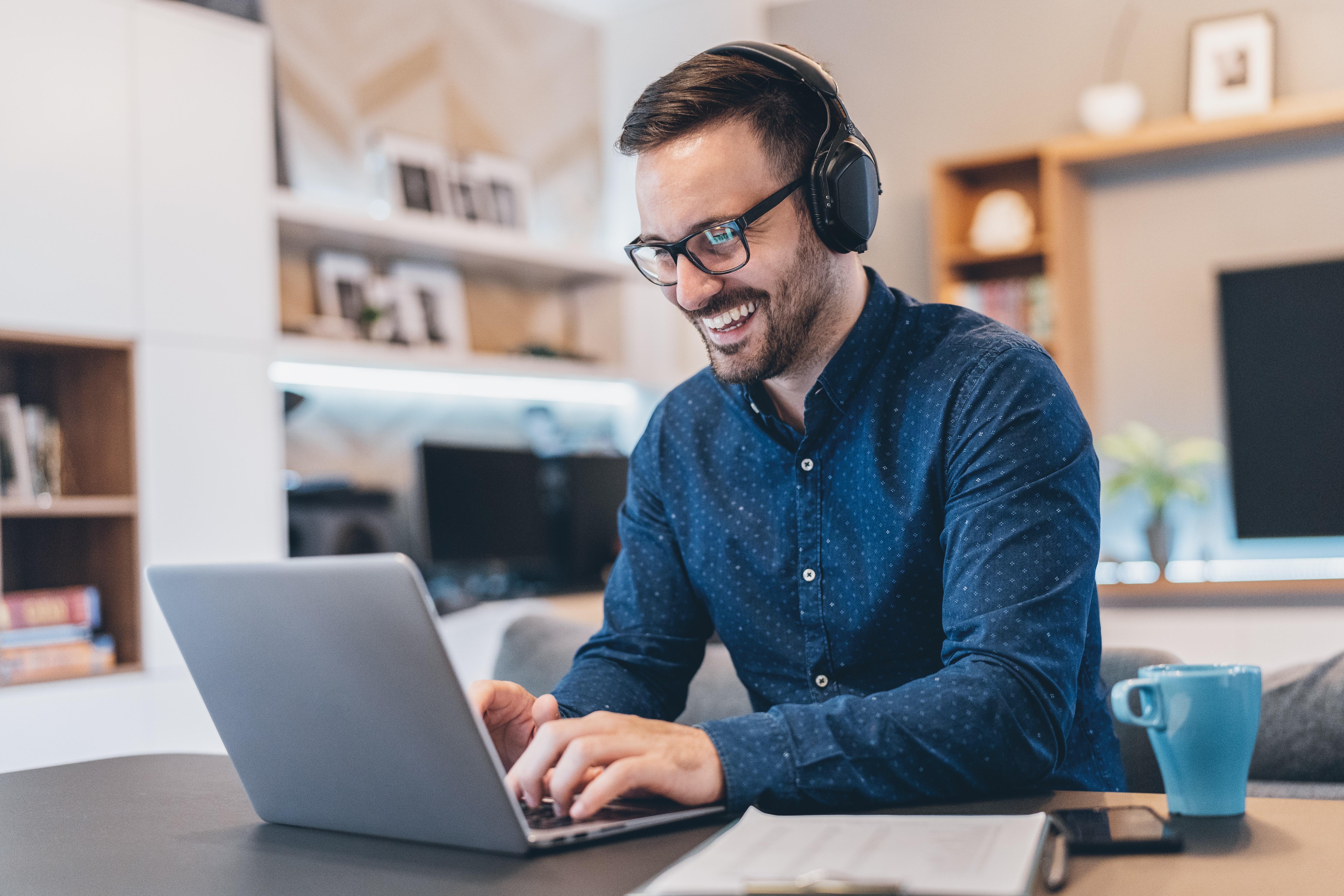
Like other sectors within the financial services ecosystem, the microfinance sector is undergoing significant changes with the rising implementation of financial technologies. Artificial intelligence (AI) is expanding the way microfinance organizations serve their clients. By digitizing their operating models through technologies like mobile banking, remote client onboarding, and predictive analytics platforms, microfinance organizations have been able to build greater resiliency and improve best practices across their value chains.
As a high-touch business, ongoing changes in this sector were likely accelerated by the pandemic. But the shift towards digital transformation was already well underway. For example, in rural areas that are plagued with poor or nonexistent infrastructure (communications, electricity, transportation), digital tools like mobile banking enables lenders to provide financial services to underserved customers. Similarly, predictive analytics tools support lenders by informing them about the risk of lending their services to a counterparty.
Strengthening ties between larger commercial banks and microfinance organizations may spur the application of financial technologies into microfinance business models. Digital opportunities like establishing digital bank ‘branches’ through mobile app development and instantaneous money transfer could provide unprecedented value to financial institutions and their clients. It’s estimated that digital innovation has the potential to unlock $60 billion in net expense reductions for banks globally by 2022. Going digital also enables banks and microfinance organizations to serve unbanked populations who cannot access traditional bank branches.
The trickier question that financial service companies and their microfinance partners have to reckon with is determining which models and technologies to adopt to meet the financial needs of the unbanked. Understanding the challenges faced by the microfinance sector and the opportunities to apply financial technologies to solve them is a key first step in this decision.
Challenges in the Microfinance Sector
The microfinance sector has continued to grow as more players enter the market and demand for financial assistance from borrowers persists, albeit at a slower rate than in previous decades. According to the Microfinance Barometer published by Convergences, between 2013 and 2018, lending by microfinance institutions (MFIs) retained an average annual growth rate of 11.5 percent, while between 2012 and 2018, the amount of borrowers slowed down to an average annual rate of 7 percent (down from the 20 percent in the prior decade). However, amidst this growth, the microfinance sector faces both internal and external challenges as it enters a new and increasingly digital decade:
- Poor banking infrastructure in rural areas
- Credit risk and overindebtedness among borrowers
Poor Banking Infrastructure
MFIs that operate in rural areas of the developing world have to navigate poor or nonexistent infrastructure to carry out financial services at scale. According to the International Labour Organization, low population density, lack of communications, electricity, and transportation infrastructure, and small loan amounts impose high transaction costs on MFIs that service individuals living in rural areas. These costs put MFIs in a precarious situation of either entering rural communities with the risk of operating at high losses, or depriving these communities from access to financial resources when they may have the greatest need for it.
The application of mobile banking (see below) could be a viable solution to introducing financial services in areas where it is too expensive to implement traditional banking infrastructure. But not all microfinance companies have fully adopted or perfected a mobile banking model in the areas they operate. As the demand for access to financial services persists, companies will have to decide whether to exit certain markets, strengthen local partnerships and increase investments to build out lacking infrastructure, or adopt digital banking.
Credit Risk
Credit risk was determined to be the greatest risk to microfinance according to “Microfinance Banana Skins 2011,” a survey of 533 participants with expertise in microfinance representing 86 countries published by the The Centre for the Study of Financial Innovation. This finding is largely due to the rise of ‘overindebtedness’ (ongoing debt) among borrowers. For example, borrowers may occasionally relocate microloans to meet their basic needs, such as food, even if they originally intended on using the credit for entrepreneurial purposes. From a lender perspective, the lender is investing in the borrower’s entrepreneurial activity and the return that it will produce. As a result, when the borrower spends the loan on household necessities instead of profitable activities, it produces a scenario where the borrower is unable to repay the loan because they are not investing in and generating additional value for their business. Oftentimes, borrowers will take out a second or third loan to pay back earlier ones, thereby trapping themselves in a cycle of overindebtedness.
Opportunity Creation in the Microfinance Sector through Digitization
Much of the halo effect around microfinance has dimmed over the past few years. Yet with the continuing wave of digital transformation penetrating the financial services industry, the microfinance sector has the potential to scale its effectiveness and reach through the implementation of digital tools. Digital transformation of the microfinance sector has been even more essential in the age of the pandemic, as global health and safety guidelines disrupted informal channels of money transfer. Here are three examples of digital tools and processes with the potential to drive greater financial inclusion and operating efficiency in the microfinance sector:
- Mobile Banking
- Artificial Neural Networks (ANNs) for Credit Scoring
- Sentiment Analysis for Credit Risk Analysis
Source: Accion
Mobile Banking
Over the last decade, the implementation of mobile banking has offered a cost-effective solution to expanding affordable financial services, particularly among rural or agricultural communities. Traditional MFIs use loan officers to facilitate financial services to borrowers, which can be costly and tedious practice, especially under circumstances where loan defaults and other financial risks are present. However, with mobile banking, microfinance customers can access financial services anywhere using their mobile devices.
Source: Center for Financial Inclusion
Most microfinance organizations do not have the capabilities to scale their services through mobile banking on their own. Instead, many have cultivated strategic partnerships with telecommunications companies (for infrastructure), commercial and investment banks (for capital), and money transfer agencies (for intermediary support) to develop a 21st century model for microfinance. By establishing an ‘agent network’ of partner mobile money outlets in rural or developing areas that lack access to ATMs or commercial banking services, MFIs expand financial services to previously unreachable individuals, such as the ability to set up accounts, withdraw and deposit cash, and take out and pay back loans.
Financial service and microfinance organizations pursuing digital banking models also have to tackle the accompanying challenge of ensuring relevant capabilities (smartphones and internet access) are available to prospective customers. To start, they’ll have to consider the risk going digital poses for customers that rely on physical branches for banking.
Using Artificial Neural Networks for Credit Scoring
Across the financial services industry, determining and mitigating credit risk requires an ongoing effort of fine tuning data extraction techniques in order to improve the reliability and speed at which financial services companies interpret a customer’s risk profile. MFIs and other financial service companies utilize credit scoring to decide whether or not a prospective client meets the criteria to receive a loan. But for MFIs in particular, making this determination is more complex since their customer base often cannot provide them with traditional financial data. Rather than relying on the five C’s of credit, MFIs can use alternative data like electronic records of financial transactions made using a mobile phone or proof of rent payment.
Automating the interpretation of this data enables MFIs to make faster (and thus, more cost-effective) lending decisions. One way MFIs can do this is by applying artificial neural networks (ANNs)—a type of machine learning (ML) model simulated after neurons in the human brain and made up of interconnected units that accept data sources as inputs.
When applied, ANNs can often provide more accurate credit scorecards than traditional methods. For example, after selecting and gathering your data (i.e. customer age, mobile phone usage, rent payments), data scientists can attribute a weight value to each input, which are then fed into a single artificial neuron. Occasionally, ANNs also contain hidden layers between the input and output units, which can support the transformation of inputs into meaningful outputs. ANN models can then be applied to predict which borrowers are creditworthy and which are not.
Source: Towards Data Science
Assessing Credit Risk with Sentiment Analysis
The set up of ANN models can be difficult to explain, which makes it challenging to work with since credit scoring methodologies have to meet certain criteria from a regulatory compliance standpoint. Another way AI can be used to understand credit risk is generating sentiment analysis from textual data through natural language processing (NLP). Sentiment analysis lets financial service companies know the overall sentiment (for example, whether something is viewed as positive or negative) of a particular company, industry, country, and more by monitoring one or multiple sources.
For example, microfinance companies that are thinking about investing or lending in an emerging market can use sentiment analysis to gain a better understanding of default rates in a specified country by monitoring the sentiment of financial news sources covering the area. However, there are a host of other ways sentiment analysis can benefit credit risk assessment processes. According to a report on textual analysis by McKinsey, sentiment analysis can be used to detect early signs of business distress for SMEs (small and medium enterprises), provide an objective analysis of an industry and its sub industries, and monitor financial-market related news for industry shifts and trends.
Using no-code AI, companies can track news and other data sources and filter by credit-related themes to extract the insights necessary to make appropriate lending and investment decisions at a client and corporate level. More importantly, for MFIs that balance business needs with social impact missions, continuously refining credit risk assessment processes is essential to stay competitive and scale their impact. MFIs that use AI tools can track and monitor large amounts of credit-related data sources (e.g. news filings, company reports) faster, which provides them with the competitive edge and cost savings to stand out in a concentrated market. From a social impact lens, making faster lending decisions enables MFIs to scale their credit services and take on more clientele because it speeds up the rate that customers are approved.
As financial service companies reevaluate the way they use financial technology to improve processes, enhance financial performance, and drive a financially inclusive future, it will be important to look out for whether the use of these tools translate to new customers and better financial outcomes for existing ones. For further reading on how AI can be used to understand, monitor, and manage credit risk, check out our blogpost on “Using Artificial Intelligence to Manage Counterparty and Credit Risk” here.
About Accern
Accern is a no-code AI platform that provides an end-to-end data science process that enables data scientists at financial organizations to easily build models that uncover actionable findings from structured and unstructured data. With Accern, you can automate processes, find additional value in your data, and inform better business decisions- faster and more accurately than before. For more information on how we can accelerate artificial intelligence adoption for your organization, visit accern.com