Building Text Analytics Models with No Code
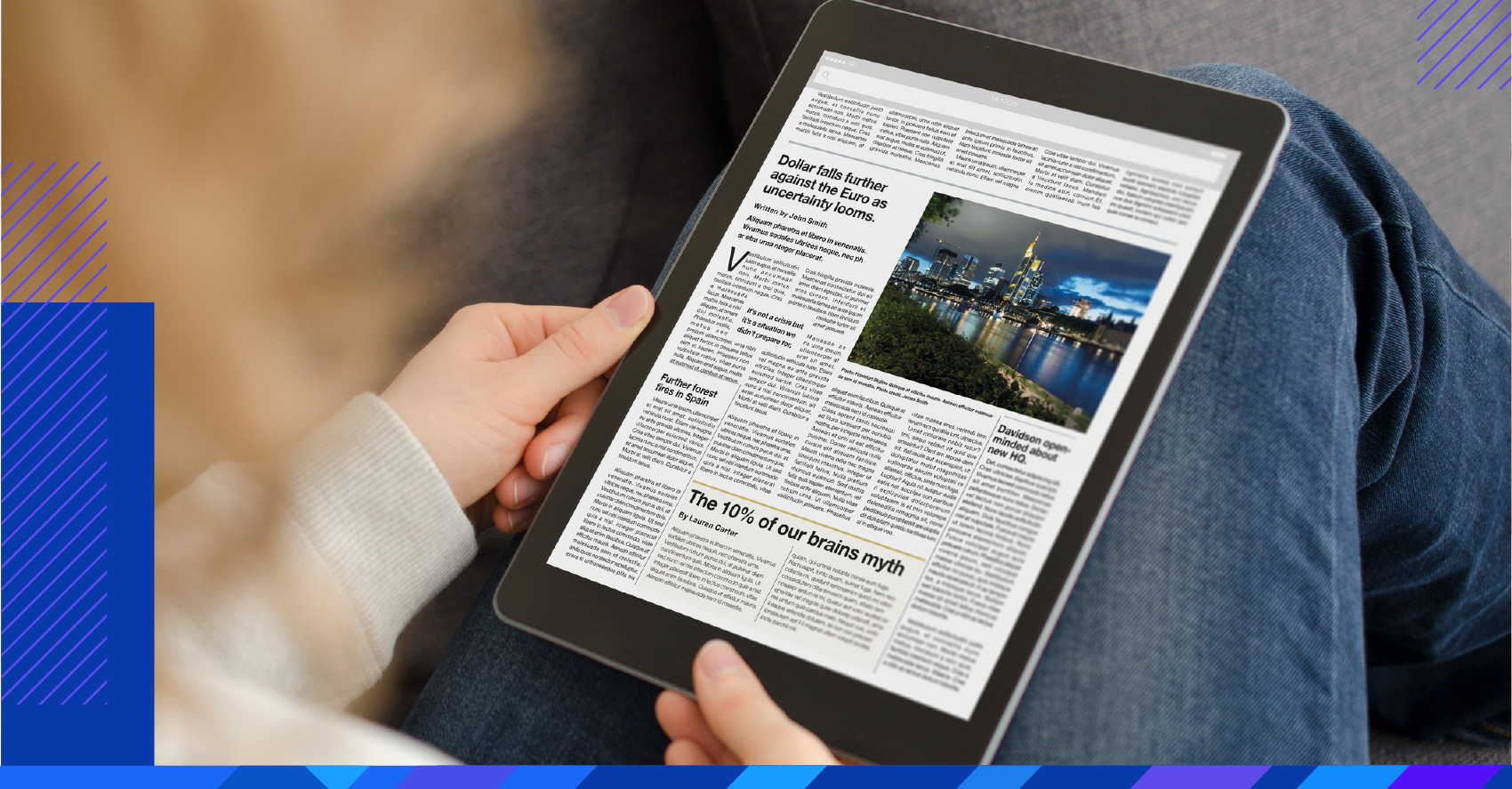
The quantity of digital text data has grown exponentially in recent years and will continue to grow. From social media posts to customer transactions, surveys, reviews, chats, emails and more, businesses face the challenges of monitoring various data sources and extracting relevant data. With innovations in AI, text analytics models can now be built on a no-code AI platform. In this blog, we'll review how users can leverage a no-code AI platform to build text analytics models that make text mining more efficient.
The rise of unstructured digital text data is an opportunity for both small and large enterprises. Along with data from new sources, businesses have found ways to generate new insights from unstructured data, leading to new text analysis software and opportunities for research.
With the rapid evolution of text analytics and with unstructured content making up nearly 80% of global data, financial enterprises have given significant attention to text mining. However, selecting the method of text analysis that generates the most ROI for an organization is an obstacle with all of the different tools available today.
Challenges of Traditional Text Mining
Source: Practical Text Mining and Statistical Analysis for Non-structured Text Data Applications by Gary Miner
Traditional text mining requires a combination of data retrieval and mining, machine learning, statistics and computational linguistics. Coding tools like Python are used to program machines to analyze text from unstructured data. Implementing the traditional text mining methods is oftentimes challenging as business leaders within financial firms must find the right text mining tool, hire subject matter experts and understand the technical aspects of it.
The first step in successfully executing a text mining approach is to ensure clean data is collected. Without reliable and high-quality data sources, financial teams will have unreliable analyses and inaccurate investment signals. Once financial teams are confident in their databases, they can then use AI to automate the process of researching and extracting data from various sources.
Modern Advances Make Developing Text Analytics Models for Text Mining Accessible
Given the structural challenges and lack of subject matter expertise on AI, financial leaders may be hesitant to invest their company's resources in AI. It can be extremely costly and time-consuming to restructure an organization so that AI can be implemented. However, with the recent advances in technology and innovation, a number of FinTech companies have emerged to bring AI to technical and non-technical users. These no-code tools make it possible for non-technical end-users to mine text and perform sentiment analysis.
Text Mining And Natural Language Processing (NLP)
Source: https://www.ontotext.com/blog/top-5-semantic-technology-trends-2017/
Text mining uses artificial intelligence (AI) and machine learning (ML) to extract and analyze information from a variety of documents. Social media, internal and external documents, emails, instant messages and articles are some of the data sources that can be used in text analytics. This process has gained popularity from AI's natural language processing (NLP) capabilities, which enable a quicker, more accurate and efficient way to research and analyze unstructured data.
NLP is a subset of AI that includes the automated process of classifying and extracting data within large sets of structured and unstructured text. It can be used to structure unstructured text and to extract the sentiment, topic, characters, relevance and intent from multiple documents. Combined with data visualization tools, text analytics and NLP can enable companies to understand the story behind their data and make better decisions.
For example, let's say you need to examine hundreds of Glassdoor reviews to understand employee sentiment around a company. With AI and ML, text-mining algorithms can extract the most popular topics from the customers' comments and analyze topics based on the sentiment — whether the comments are positive, negative or neutral. Additionally, you can identify keywords regarding a given topic for insights into the company and its products and services. In a nutshell, text mining allows teams to analyze raw textual data on a large scale and extract actionable insights.
AI has transformed the way that financial teams work as firms recognize the productivity gain and revenue benefits of implementing AI into their teams' workflows. The global AI market in FinTech is expected to hit $22.6 billion in 2025. Now with technological advances, text mining is more accessible to end-users with different technical capabilities.
No-Code NLP for Text Mining
Source: Accern NLP Models
No-code NLP tools powered by AI now provide ready-made and powerful cloud-based use cases. Researchers and analysts can select from pre-trained NLP models to yield specific finance solutions. Accern offers a number of ready-made use cases that provide specific solutions to each finance industry's problems. For example, asset management firms and banks can use our use cases on credit risk and ESG, while insurance firms can use our AI for underwriting and detecting fraud.
Users are often misled by the idea of a "ready-made" use case, as they often believe that customization is limited. However, that's not always the case. Accern's ready-made use cases can act as a "quick start" tool and can be retrained. NLP models can be retrained through an intuitive, no-code NLP model trainer to generate custom insights quickly and efficiently.
SaaS platforms offer the clean data required for a successful text mining approach. Users can either import their datasets from emails, company documents, and CRM systems into the platform, or access integrated datasets from external providers.
In other words, no-code AI tools change the game for text mining and predictive analytics. However, keep in mind that not all companies are fit to implement AI and ML. Companies that do not store large volumes of data internally or require data processing from external systems will most likely not find significant benefits from AI.
Is No-Code AI Right For Your Organization?
When considering no-code AI, we ask our customers to consider these five questions:
- What business process can be enhanced with automation?
- What type or types of data are connected to the use case?
- Is the data located in a place where an AI process can see it (e.g., a CRM and CMS systems, Google Analytics, imported documents, etc)?
- What type of insights are you looking to draw from the data?
- How will the insights be consumed (e.g., through an API, a dashboard, etc.)?
These questions can help clarify whether no-code AI is right for your organization and create a strategy around how no-code AI can be used to maximize work efficiency. A successful AI implementation strategy includes identifying the structural components of bringing AI into an organization, the areas of improvement, setting clear business and technical objectives, and ensuring a process for continuous improvement to understand how AI can fit into the corporate DNA.
About Accern
Accern is a no-code AI platform that provides an end-to-end data science process that enables data scientists at financial organizations to easily build models that uncover actionable findings from structured and unstructured data. With Accern, you can automate processes, find additional value in your data, and inform better business decisions—faster and more accurately than before. For more information on how we can accelerate artificial intelligence adoption for your organization, request your free demo below.
Request a Demo