Bias In AI: New Perspectives on Detection and Reduction
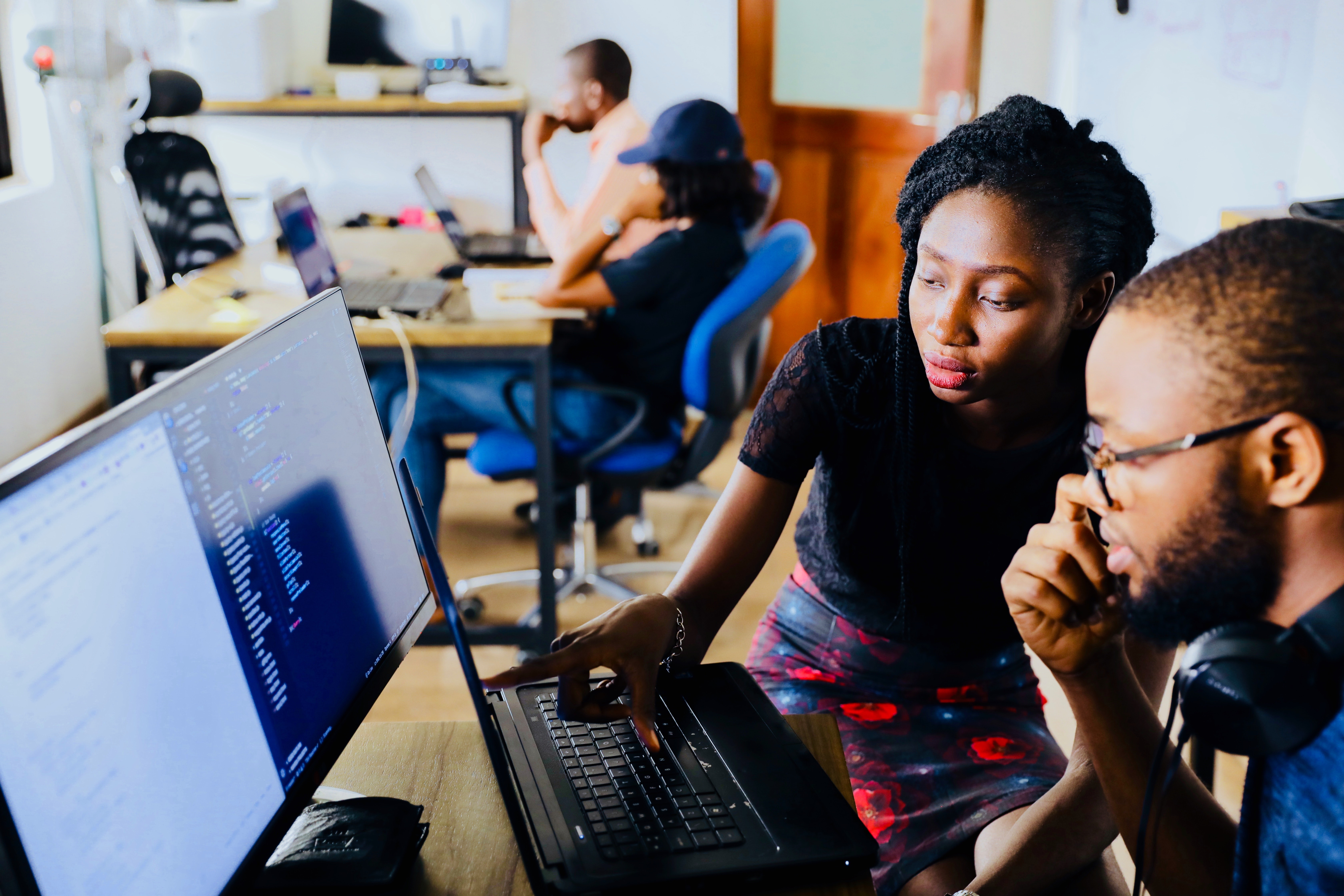
As global businesses open the books in 2022, more than half have listed the pandemic as an accelerator behind the adoption and use of artificial intelligence (AI). Dig a little deeper into that report from data aggregator Appen and you’ll find a somewhat surprising emphasis, 80 percent of all business leaders in fact, that list data diversity and bias reduction as either “very” or “extremely” important. So in addition to continuing to scale AI usage, reducing bias in its algorithms is one of the key challenges of this year.
More than any other industry, financial services organizations need to navigate the issue of bias carefully. As it continues to become more accessible and applicable, artificial intelligence has served financial institutions well. In fact, 75 percent of banks holding above $100 billion in assets are already using AI for applications ranging from loan and credit decisions to risk assessment and tracking market trends. But as always, there’s room for improvement for banks to use AI. As it is used more often, a “ghost in the machine” is becoming more apparent: bias.
Bias in AI programs is akin to a virus in a software system. Because bias can affect data quality it can reduce the accuracy of algorithms in an industry where data science needs to be exact. Bias can also be a limiting factor as banks reach out to more customer segments, including those who have been outside the financial system or have not been able to achieve a traditional credit score. Although biases exist in several areas of the AI process, through human and automated reforms they can be identified and reduced. This blog post discusses how to detect and reduce AI biases.
But first, let’s take a look at the stakes in this mission. AI ethics are involved, as financial services deal with the controversial issue of extending more products to lower-income groups. The problem has been big enough to attract the House Of Representatives Financial Services Committee. It started in a late November letter to the Federal Reserve that “any use of AI in the financial services industry must emphasize principles of transparency, enforceability, privacy and security, and fairness and equity, with strict scrutiny on financial institutions that exhibit algorithmic bias or engage in technological redlining. AI must be used in a way that serves the American public, its consumers, investors, and labor workforce, first and foremost.” Note that the letter addresses the human element much more than the technology behind AI.
The Human Element:
As stated in a recent MIT Technology Review article, the “human filter makes all the difference in organizations’ AI-based decisions.” It’s the key to understanding how bias shows up in AI algorithms. Humans create algorithms and algorithms create models. The first step in detecting bias starts with the beginning of the equation: humans.
Most psychological studies have identified 180 different human biases, some conscious, some unconscious. When humans (either individually or as a team) create the data set that will eventually become an algorithm, any one of those 180 biases from clustering to overconfidence can find its way in. Here are four biases that can have the biggest negative impact on identifying actionable projects, aggregating the right data and creating bias -free algorithms.
Reporting: This bias occurs when only a selection of results or outcomes are captured in a data set. For example, if a bank in California is building an algorithm to predict identity fraud and it neglects to include data from less populated states, that data will be biased toward population-intense areas and could therefore misrepresent the complete picture of ID fraud.
Selection: Data science teams sometimes narrowly focus on solving a specific problem without considering how the data will be applied. This can lead to selection bias, which occurs when teams select a participant pool, such as mortgage holders for example, that is not representative of the target population.
Attribution: When teams generalize individual data to an entire group, attribution bias is a common result. If that mortgage holder group is shown to have a high propensity of delinquency among millennials, it doesn’t mean all millennials are financially irresponsible.
Prejudicial: This has the potential to show up when teams inject a widely held stereotype against certain groups. Example: If inner-city loan applicants are routinely rejected because those types of regions have a lower income level, the data is biased
Detecting bias
Once financial services organizations understand what makes up AI bias, they can then detect it. As detailed in Stanford’s Social Innovation Review, companies can detect bias if the results suffer from faulty comparisons. “No matter how sophisticated, predictive algorithms and their users can fall into the trap of equating correlation with causation,” it said. “In other words, of thinking that because event X precedes event Y, X must be the cause of Y.”
Some of those results can be obvious. For example, if a credit evaluation algorithm’s average score for male applicants is significantly higher than it is for women, further investigation and simulations could be warranted. It’s more than likely the data was biased. Less obvious comparisons can also raise a red flag. For example, running algorithms alongside analysis by human decision-makers, and then comparing them, could explain possible biases.
Other ways to detect bias can be seen in unintended consequences of the results. AI algorithms, if biased, could present actions that are against privacy statutes or local compliance protocols. In the EU, banks have run up against this as they relate to the strict privacy regulations in that region. Finally, irrelevant KPIs is a bright red flag. If the bias has worked its way through the algorithm and into the AI model it’s possible that the KPIs generated will not be relevant to the original intent. For example, if a bank uses an AI program to identify fraud patterns and finds the percentage of fraudulent transactions is non-existent, something is wrong with the data.
Fixing The Bias Problem:
Remember that biases start with humans. It’s with humans that the most important bias mitigation can be achieved. Combining that human element with solid data science either internally or with a partner is a powerful combination.
Redefine the business problem:
For example, a human-centric approach to reducing AI bias can be to redefine the business problem the team initially set out to solve. Trying to solve too many scenarios can be unmanageable. Narrow the problem and you mitigate the risk of bias. This can be achieved simply by asking the right questions: does the data expected to accomplish a business goal? Can the business activities on the data when it’s received? Define benchmarks for success even before the project starts.
Retraining data
From a data perspective, many experts have pointed to “re-training” the original data inputs. Biased AI models usually need more data points to reduce discrepancies, which is where the “re-training” term comes from. New techniques such as transfer learning are making that possible. Transfer learning is a simple concept on the surface. A mechanic who works on car engines, for example, will be able to recognize the same structures when transferring to trucks.
In the AI context transfer learning means that good data can stay in the equation. If bias is found, the entire algorithm doesn’t need to be scrapped. So “re-training” doesn’t mean “restarting.” For example, if a brokerage creates an algorithm that over indexes (or is biased) in favor of high wealth clients, the basic data can be supplemented and then transferred to a new model working in a new environment.
How No-Code AI is Transforming, Detecting, and Reducing Bias
No-code AI is built perfectly to detect and mitigate bias in the financial services industry. By using a no-code approach, retraining data, which is an essential mitigation strategy, can be done without disrupting the entire data science team. No-code AI easily integrates new data sources, and as a result, the bias that often creeps into algorithms can be easily remedied. As we’ve seen bias is both a human and data issue, and no-code AI lets the humans balance out the equation. For more information on no-code AI’s ability to detect and reduce bias, request a demo today.