3 Use Cases for NLP in Insurance
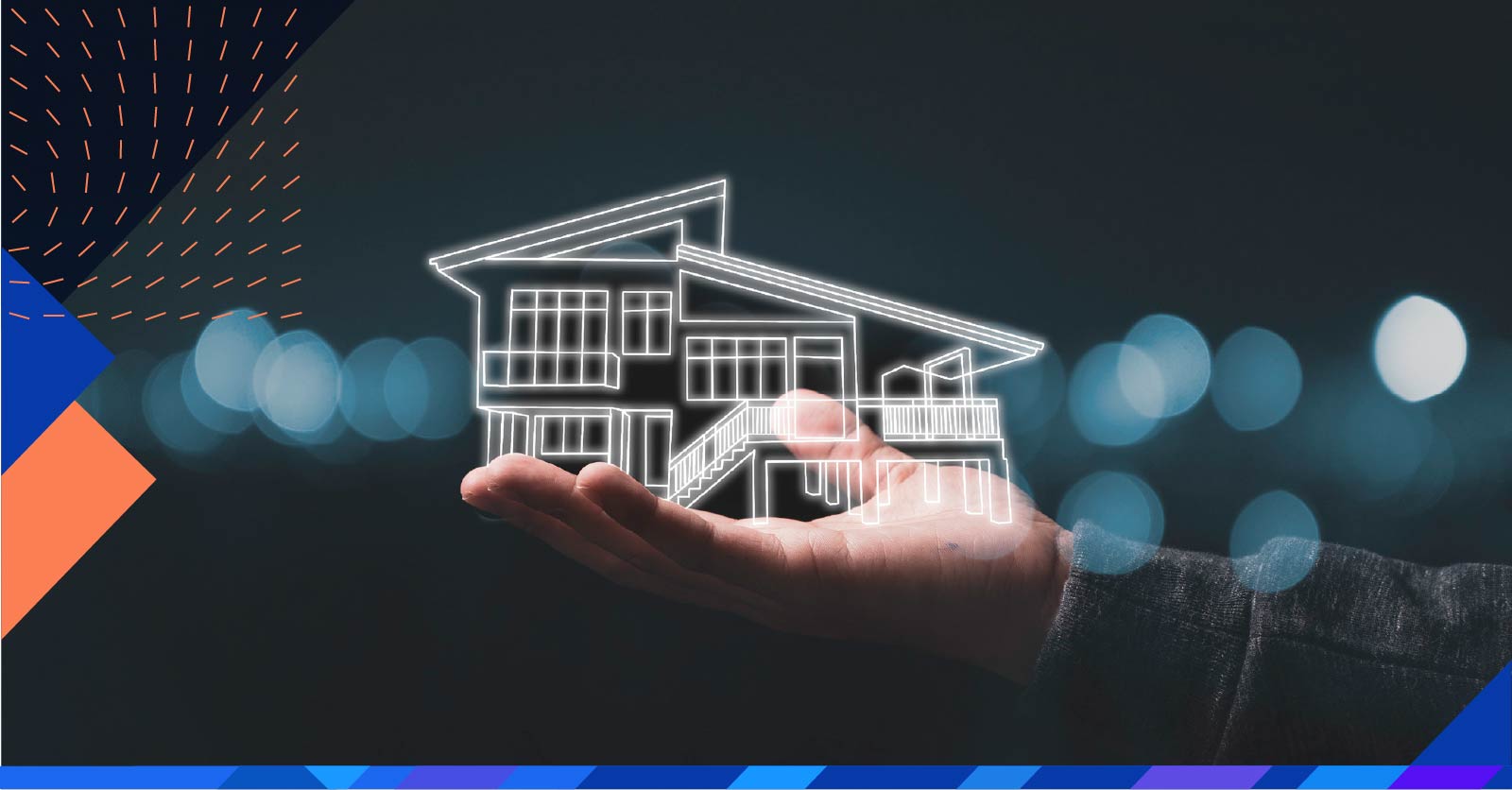
Insurance is a valuable industry, with insurance premiums written in the United States recently reaching over a trillion dollars. Despite the large sums of money at play, the industry has still been slow to adopt technological advancements due to the nature of the business and its strict regulations.
Insurance firms are still beginning to see all AI and ML use cases to improve and modernize operations. Among all the ways financial services firms adapt to emerging technology, AI—and specifically NLP in insurance—is becoming increasingly common. Firms utilize AI's powerful automation and data processing capabilities to make their front and back-end workflows more efficient, improve the customer experience, and save valuable time and money.
What are NLP Use Cases?
Within AI and ML, insurance firms implement natural language processing (NLP) to extract meaning and actionable insights from text data. NLP is a fast-growing sub-segment of AI taking hold in the financial services sector, allowing for smooth and natural human-machine interfacing.
There are countless ways for NLP to be applied to a business's operations. For example, NLP can decipher and classify language-based documents, interact with customers via chatbots or voice assistants, interpret and scan news articles for relevant information, or even translate text data from one language to another.
More specifically, NLP use cases in insurance continue to evolve and provide a way for these firms to become more efficient and accurate in their solutions. Expanding a company's risk management capabilities, fraud detection, and improving customer service are vital ways insurance companies utilize NLP. However, there are numerous other applications for NLP within these firms.
AI's Impact on the Insurance Industry
For an insurance company, the amount of information that needs to be processed each day to assess the risk profiles of new clients, determine premiums, and process claims becomes too much for human workers alone. Given the capabilities of AI to accurately and efficiently analyze large amounts of data each day, this tool is revolutionizing the space and helping these firms save valuable time and money at every stage.
Additionally, more data is available on consumers than ever before, but it comes from various sources and is not always standardized or available in centralized locations. While this would be tedious and time-consuming for staff to compile all relevant documents and data points on a client from each source, AI can streamline this process. By filtering and sorting through several databases and sources to extract information, AI can work around the clock to keep insights up-to-date.
3 Use Cases for NLP in Insurance
As NLP use cases in insurance continue to expand and transform the space, firms can find many practical applications of this technology in their operations. By providing valuable insights into customer behavior, facilitating better prediction capabilities, and helping firms become more efficient, NLP is changing the landscape of the insurance industry and how these firms operate and deploy resources.
1. Obtaining Individual and Corporate KYC Information
In 2012, Rule 2090 into place by FINRA, which requires financial institutions to make reasonable efforts to know and keep records on essential customer information. Better known as KYC data, or know your customer, this regulation was put in place to protect these firms from fraud and corruption and prevent them from unknowingly facilitating illegal activities through their organization.
Each new regulation in the industry adds to these firms' already hefty and backlogged workload. Therefore, using NLP in insurance can significantly help these firms meet the due-diligence requirements of this rule. NLP models can automatically sort, file, and extract meaning from thousands of documents and records for each individual or corporate customer and compare them against historical records to determine if their activities and funds are legitimate.
NLP is helping insurance companies extract meaningful data from propriety and third-party sources to quickly learn the basic information about a customer, which would take a significant amount of time for employees to do manually. In addition, NLP helps with the compliance aspect, keeping all relevant files and documents sorted, filed, and easily accessible.
2. Customer Credit Risk Alerts
With NLP, insurance companies can receive real-time alerts around the credit risk of their clients, using the most up-to-date data. This has significant implications for insurance firms, as risk management is the basis of their business model. Plus, the mountains of data that would need to be processed by personnel can not be done in real-time.
The more efficient insurance companies can access their customer's risk profiles, the less risk they will be exposed to as a firm, and the quicker they can make informed decisions regarding their coverage. NLP models can assess risk from more than just the firm's proprietary data–they can use third-party sources to understand the customer's complete risk profile better.
Overall, using NLP in insurance helps firms become more agile and responsive to data in real-time. Take an auto insurance firm; say their customer has gotten into an accident. The firm could adjust its monthly premium to make up for the increased risk. Thus, enhanced risk management is one of the significant NLP use cases in insurance.
3. Claim Identification
A high cost to insurance companies comes from fraud, primarily through false or fraudulent claims. It was recently estimated that these activities cost insurance companies in the United States $80 billion each year, so it is meaningful for these firms to find better and more accurate ways to detect a suspicious claim.
Applying NLP in insurance can help firms assess historical data and compare it against current claims being made, recognizing patterns in claims and flagging outliers for investigation. Processing claims has traditionally been a tedious and time-consuming task, and each worker may not be able to notice distinct patterns or questionable claims when manually processing them each day.
With powerful predictive analytics capabilities, NLP models can provide early detection of potentially fraudulent claims and be aware of which customers are more likely to make false claims in the future. With the potential to save insurance firms billions of dollars each year, integrating NLP into a firm's workflow can save claims agents hours that can be spent on other activities, prevent losses, and reduce the human error that is prone in these types of tasks.
The Impact of NLP on Insurance
As there continues to be a growing number of NLP use cases in insurance, the firms that have traditionally avoided emerging technology are beginning to see all the ways these models can benefit their workflows and improve efficiencies. Through better risk management, enhanced fraud detection, and quicker data processing capabilities, using NLP in insurance helps firms comply with obligations and become more responsive to emerging information that could save them billions.
How No-Code NLP Helps Insurance Firms
While the insurance industry begins to embrace recent technological advancements in a way it hasn't done before, the use of AI and ML in these firms will continue to rise. Saving firms valuable time and money, the sub-segment of NLP is helping firms extract meaningful insights from text data and operate at all hours of the day to provide real-time updates.
With the use of a no-code NLP solution, like the one offered by Accern, any insurance firm can easily access these ready-made models that can quickly integrate into their current workflows and technology stacks. Financial professionals who understand these complex technologies can quickly implement them into their daily processes without coding. Sign up for a free trial today to see how your firm can reap the benefits of these powerful AI models.